Event Details
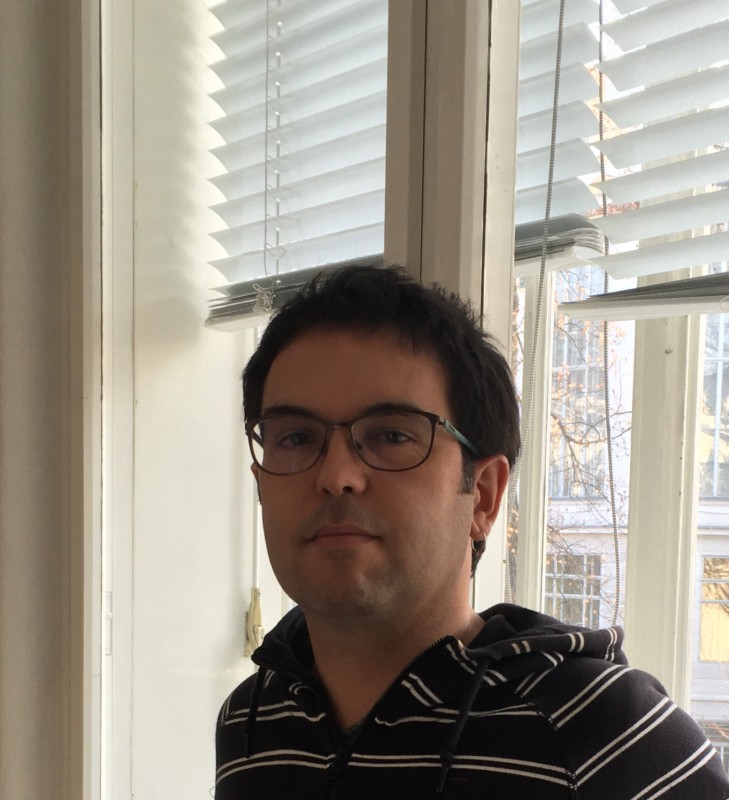
Topic description:
A major problem for evolutionary theory is understanding the so called open-ended nature of evolutionary change, from its definition to its origins and consequences. Open-ended evolution (OEE) refers to the unbounded increase in complexity that seems to characterise evolution on multiple scales. This property seems to be a characteristic feature of biological and technological evolution. OEE represents a tremendous challenge for our attempts to develop a ‘theory’ of evolving systems —maybe makes it impossible, in standard terms—, and calls for a deep revision of concepts such as ‘prediction’ or ‘law’. In this talk we will present a fundamental characterisation of OEE based on Algorithmic Information Theory, which can be understood as postulates for the OEE. Essentially, such postulates assume intrinsic unpredictability and the need for an always increasing amount of information to explain the successive evolutionary steps —the emergence of innovation. Interestingly, such unpredictability defines the boundary conditions for a mathematical problem which ends with a prediction: The statistical counterpart of the OEE postulates, based on standard Shannon Information theory, has the structure of a variational problem which is shown to lead to Zipf’s law as the expected consequence of an evolutionary processes displaying OEE. Interestingly, many complex systems candidates of displaying OEE, from language to proteins, share this common scaling behaviour. We therefore have been able to predict a global pattern, although we lost the Newtonian or Lagrangian determinacy, because we ignore which kind of elements will populate our space of possibilities: The concept ‘prediction’, therefore, must be dissected and the potential laws appearing for OEE systems may be of different nature than the ones displayed in physics. We will further revise the problem of information conservation through an OEE process and we conclude that standard Shannon information is not conserved, resulting into the paradoxical situation in which the increase of information content has the effect of erasing itself. We will prove that this paradox is solved if we consider non-statistical forms of information. This last result implies that standard information theory may not be a suitable theoretical framework to explore the persistence and increase of the information content in OEE systems. Concepts such as ‘natural law’, ‘prediction’ or ‘information’ unfold in several ones when non-standard assumptions are done, such as in the case of OEE, and they must be critically revised.
Biographical note:
Bernat Corominas-Murtra has a degree in Physics by the Universitat Autònoma de Barcelona, a degree in Linguistics by the Universitat the Barcelona, and a master in Mathematics by the University of Barcelona. He got his PhD at the Complex Systems Lab/ Universitat Pompeu Fabra (Barcelona) under the advise of Ricard Solé. In 2013 he joined The Section for the Science of Complex Systems of the Medical University of Vienna, headed by Stefan Thurner. His past works span from complex networks to information theory and interdisciplinary approaches to biological problems, brain dynamics or human language. Currently he is working in the study of path-dependent stochastic processes and developing generalised forms of Information Theory and statistical mechanics which go beyond the scope of classical approaches to embrace the phenomenology associated with evolving, complex systems. The main aim of his research is to provide a solid theoretical background for path-dependent and evolving systems and for the emergence of complexity associated to them.